How to Test and Ensure Highest Data Quality
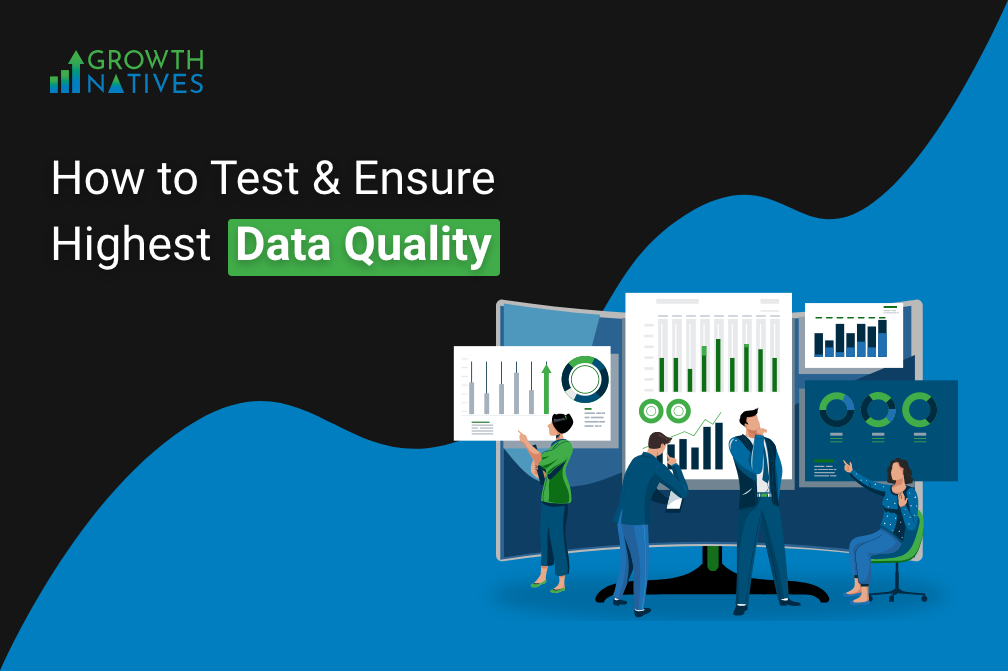
Table of Contents
“The goal is to turn data into information and information into insight.” – Carly Fiorina, former CEO of Hewlett Packard.
Data is the core of any business today. The quality of data collected, stored, and processed determines the success of your business operations. Despite knowing what data can do for a company, people are unable to understand the importance of “data quality”.
In this article, we are going to discuss data and data quality and uncover common questions like how to measure data quality, so keep on reading. But first… we need to understand what DATA QUALITY is.
What Is Data Quality?
Data quality defines the condition of data gathered determined by various factors such as consistency, relevance, reliability, completeness, and if it is in sync with the trends.
So, if the gathered data helps in running smooth business operations and making an informed decision that shows positive results, then you have good quality data.
Now that you know what data quality is, let’s disperse some common misconceptions about quality.

We understand that bad data can jeopardize your business operation, but it is difficult to gather perfectly accurate data – and this is one of the biggest misconceptions among companies. They believe that data quality means having error-free data. But that is not the case. With tools and campaigns being used to collect piles of data, getting error-free data is impossible.
Thus, to have the right quality data, it is important to have three things in place:
- What are the data collection requirements?
- How are these requirements created?
- How much leeway do we have to meet these requirements?
Once you have a clear understanding of these questions, it will be easy for you to determine data quality.
Remember, gathering data to use it for decision-making requires companies to be open to potential risks and errors. Having zero-error data is nearly impossible to achieve and cam also scarcely moves the ROI pointer.
This is the reason, data quality plays a crucial role here – it is a perfect balance for understanding data accuracy and comprehensiveness.
Why Is Data Quality Important?
Bad data will have a substantial impact on a company's bottom line. Oftentimes, poor data is attributed to operational blunders, erroneous analytics, and terrible business decisions.
Moreover, bad data quality can result in added expenses, lost customers or sales opportunities, and sometimes companies have to pay huge compensation for inadequate economic or regulatory compliance.
Gartner’s survey shows that companies have to pay an average of $15 million annually for bad data in 2017.
Uber stated in 2017 that its accounting system miscalculated its commission cut, resulting in the underpayment of drivers. The issue was caused by an upgrade to Uber's terms of service in 2014.
A survey by Harvard Business Review states that only 3% of companies meet the good data quality norms.
This shows that good data is the need of the hour today. With this in place, the difficulty to convince everyone in a company that having good data quality is beneficial is no more there. The consensus for focusing on data quality is considerably stronger in this era of digital transformation than it was ever before.
Data Quality Checklist
From websites to marketing campaigns, companies are spending time and resources to collect data – lots and lots of data – which makes it important to have a checklist in place that ensures you are working on the right and best quality data.

- Accuracy – It should show how well data represents the real-world entity.
- Uniqueness – The data is one of its kind when it is measured against other entities.
- Completeness – The data should have all the needed values; any missing records will make it hard for decision-makers.
- Timeliness – It should be up to date, whether it is about previous sales or any other information.
- Consistency – The data gathered should be based on the pre-conceived patterns and cross-referenceable with the same outcomes.
- Validity – The data gathered should be based on the requirements given.
Depending on the demand and the type of data, the criterion for acceptable data quality might vary. Remember, data quality and accuracy are not about “one size fits all.”
As more prospects enter and new markets are established, the data will continue to go up, therefore there will never be an "optimal moment" to address data quality concerns. Getting started is by far the most crucial step you can take today.
Maintaining Data Quality
Data Quality Dimensions
The goal will be to assess and enhance a variety of data quality aspects while improving data quality. Data redundancy, which means two or more database rows describing the same real-world entity, is common in customer master data, which is why uniqueness is the most crucial factor to evaluate the data quality dimension.
Other data quality dimensions to measure and improve are - data accuracy, which refers to real-world alignment with a valid source, data validity, which indicates that data meets the specified operational requirements, and data integrity, which indicates that relationships between entities and attributes are consistent.
Data Quality Tools
Data quality tools are processes to identify, understand, and correct data issues to meet the effectiveness of information that facilitates business processes and informed decision-making.
Simply put, data quality tools assist you in implementing data quality management (DQM) in your company. Because these technologies are so critical, you must guarantee that they thoroughly handle all of your problems, from identifying the issue to resolving it and managing procedures to prevent a recurrence.
Data Cleaning
It's the process of deleting inaccurate or duplicate entries while also correcting any suspicious or missing data. The data quality tool comes in handy in detecting and correcting such errors.
Data Profiling
Data profiling helps in understanding the data resources associated with data quality management, which is frequently supported by advanced technology.
The prevalence and distribution of data values on structural levels are tallied during data profiling. It can be used to assess data integrity explicitly or as a starting point for assessing additional data quality metrics.
Best Practices For Successful Data Quality
- Address Data Pipeline
Finding and addressing existing errors in data sets should not be the exclusive emphasis of data quality. Instead, companies should concentrate their efforts on the whole data pipeline, seeing data quality as a continuous process that starts the instant when a new data element is entered. Data quality initiatives should be developed to address every step of the data pipeline in a proactive manner.

For example, when a user enters your website and downloads your ebook or replays a video guide, it should be immediately detected and gathered. Waiting until after risks the efforts for sales to follow up.
- Stay One Step Ahead
Data is being used by businesses to bring value to their business operations and strategic choices more than ever before. However, the majority of large businesses are already aware that poor data quality is endangering their efforts to use data as a strategic advantage.
For example, contact information is not always feasible and is sometimes understood as bad data because leads can change their address, location, company, and contact information. Moreover, human errors can also drastically impact the findings.
With data quality practices, businesses can stay ahead of the problem by taking preemptive measures to detect and address problems and institute steps to prevent errors from emerging beforehand.
- Implement Data Governance Initiatives
Data governance is more than just following the regulations and keeping your data secure. Data governance is defined as a set of procedures, regulations, and KPIs that assure an organization's ability to fulfill its objectives through the efficacious use of information.
Companies that are struggling with data quality should have data governance policies in place. In addition, the data governance model must include the organizational structures that are required to achieve the desired degree of data quality.
- Understand Data Requirements
One of the most essential aspects of high data quality is meeting requirements and delivering data to end-users for the purposes for which it was created.
However, precisely presenting the data is difficult. To truly grasp what a client wants, you'll need to go deep into the data, understand it, and communicate it coherently - this can be done by using data examples and visuals.
If all of the dependents or criteria aren't examined and documented, the requirement is termed unfulfilled, which is why to capture all the needed data conditions and requirements.
- Leverage Cloud Solutions
Decision-makers all across the world use data from a variety of sources and places, both on and off the business network. If your data quality tools are housed in only one or two company data centers, it will be difficult to get consistent data from different sources to business analysts.
Having a cloud-native solution in place will result in the high availability and suppleness of data. Furthermore, it decreases the burden of server maintenance, configuration, data access, updates, and other technical requirements.
Wrapping Up
Working with data has its own challenges. However, by monitoring data quality at every step and using the right data quality management tools it is feasible to keep data in order - right from the stage data is imported into your systems to using it for decision-making and business operations.
If you are looking for a data quality tool or need a team of experts to help you understand data quality, do connect with us at info@growthnatives.com.
Author Box
Sakshi Arora
Sakshi Arora is a seasoned content writer and editor with extensive experience across various industries including B2C, B2B, travel, e-commerce, and IT. In her free time, she enjoys expressing her creative side through painting and writing poetry. She also finds solace in nature and has a deep spiritual connection. Music brings her immense joy.